Document Type
Article
Version Deposited
Published Version
Open Access Funding Source
Open Access Publishing Fund
Publication Date
4-10-2021
Publication Title
BMC Bioinformatics
DOI
10.1186/s12859-021-04113-8
Abstract
BACKGROUND: The nucleus of eukaryotic cells spatially packages chromosomes into a hierarchical and distinct segregation that plays critical roles in maintaining transcription regulation. High-throughput methods of chromosome conformation capture, such as Hi-C, have revealed topologically associating domains (TADs) that are defined by biased chromatin interactions within them.
RESULTS: We introduce a novel method, HiCKey, to decipher hierarchical TAD structures in Hi-C data and compare them across samples. We first derive a generalized likelihood-ratio (GLR) test for detecting change-points in an interaction matrix that follows a negative binomial distribution or general mixture distribution. We then employ several optimal search strategies to decipher hierarchical TADs with p values calculated by the GLR test. Large-scale validations of simulation data show that HiCKey has good precision in recalling known TADs and is robust against random collisions of chromatin interactions. By applying HiCKey to Hi-C data of seven human cell lines, we identified multiple layers of TAD organization among them, but the vast majority had no more than four layers. In particular, we found that TAD boundaries are significantly enriched in active chromosomal regions compared to repressed regions.
CONCLUSIONS: HiCKey is optimized for processing large matrices constructed from high-resolution Hi-C experiments. The method and theoretical result of the GLR test provide a general framework for significance testing of similar experimental chromatin interaction data that may not fully follow negative binomial distributions but rather more general mixture distributions.
Recommended Citation
Xing, H., Wu, Y., Zhang, M.Q. et al. Deciphering hierarchical organization of topologically associated domains through change-point testing. BMC Bioinformatics 22, 183 (2021). https://doi.org/10.1186/s12859-021-04113-8
Creative Commons License
This work is licensed under a Creative Commons Attribution 4.0 International License.
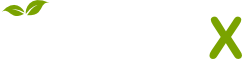
- Citations
- Citation Indexes: 12
- Usage
- Downloads: 63
- Abstract Views: 4
- Captures
- Readers: 18
- Mentions
- Blog Mentions: 1
Comments
Article Processing Charges making this article Open Access were supported by the 2020-21 Rowan University Libraries Open Access Publishing Fund.